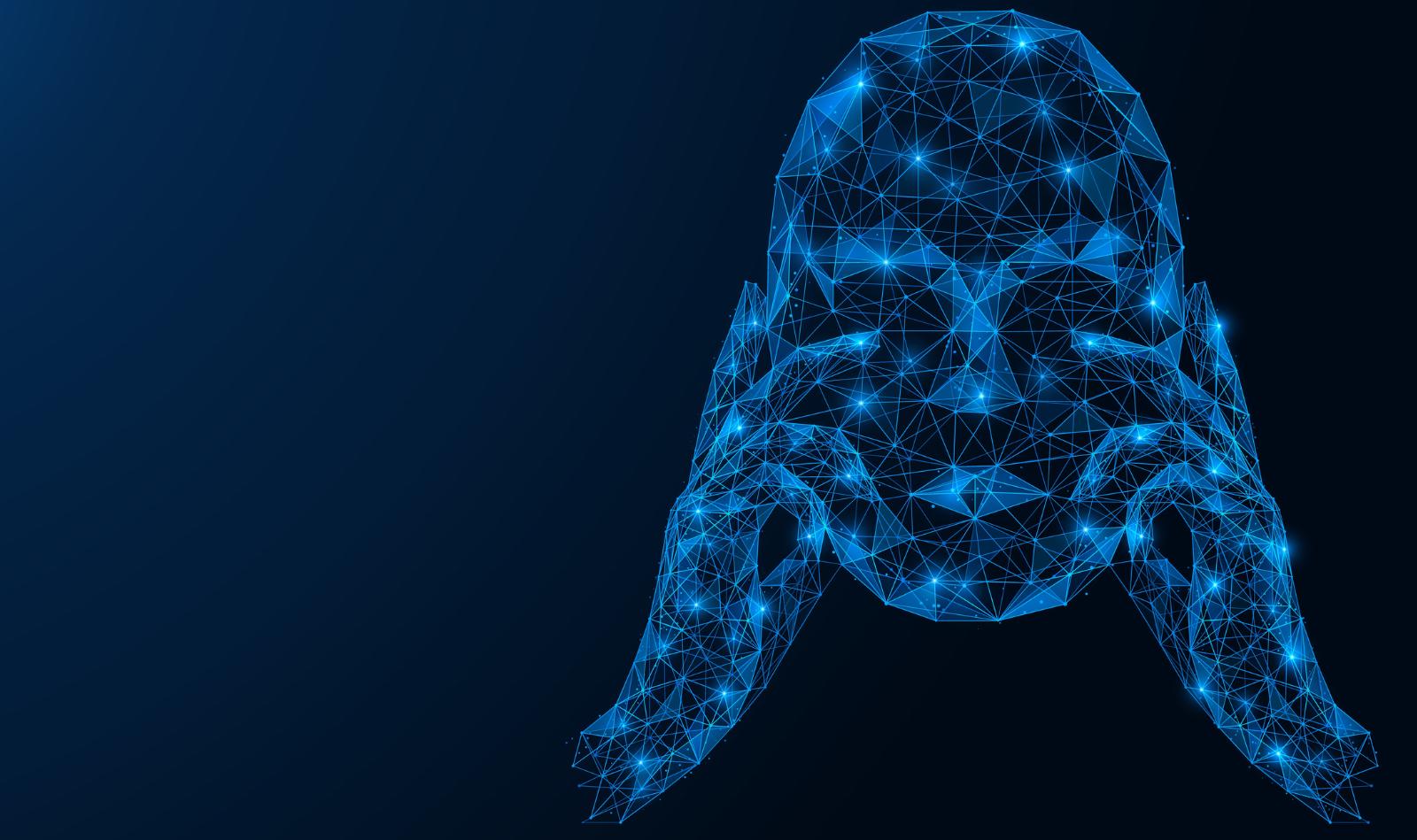
The Great Pretender
Reading Time: 5 minutesThere is a good reason not to trust what today’s AI constructs tell you, and it has nothing to do with the fundamental nature of intelligence or humanity, with Wittgensteinian concepts of language representation, or even disinfo in the dataset. All that matters is that these systems do not distinguish between something that is correct and something that looks correct. Once you understand that the AI considers these things more or less interchangeable, everything makes a lot more sense.
Now, I don’t mean to short circuit any of the fascinating and wide-ranging discussions about this happening continually across every form of media and conversation. We have everyone from philosophers and linguists to engineers and hackers to bartenders and firefighters questioning and debating what ‘intelligence’ and ‘language’ truly are, and whether something like ChatGPT possesses them.
This is amazing! And I’ve learned a lot already as some of the smartest people in this space enjoy their moment in the sun, while from the mouths of comparative babes come fresh new perspectives.
But at the same time, it’s a lot to sort through over a beer or coffee when someone asks ‘what about all this GPT stuff, kind of scary how smart AI is getting, right?’ Where do you start — with Aristotle, the mechanical Turk, the perceptron or ‘Attention is all you need’?
During one of these chats I hit on a simple approach that I’ve found helps people get why these systems can be both really cool and also totally untrustable, while subtracting not at all from their usefulness in some domains and the amazing conversations being had around them. I thought I’d share it in case you find the perspective useful when talking about this with other curious, skeptical people who nevertheless don’t want to hear about vectors or matrices.
There are only three things to understand, which lead to a natural conclusion:
- These models are created by having them observe the relationships between words and sentences and so on in an enormous dataset of text, then build their own internal statistical map of how all these millions and millions of words and concepts are associated and correlated. No one has said, this is a noun, this is a verb, this is a recipe, this is a rhetorical device; but these are things that show up naturally in patterns of usage.
- These models are not specifically taught how to answer questions, in contrast to the familiar software companies like Google and Apple have been calling AI for the last decade. Those are basically Mad Libs with the blanks leading to APIs: Every question is either accounted for or produces a generic response. With large language models the question is just a series of words like any other.
- These models have a fundamental expressive quality of ‘confidence’ in their responses. In a simple example of a cat recognition AI, it would go from 0, meaning completely sure that’s not a cat, to 100, meaning absolutely sure that’s a cat. You can tell it to say ‘yes, it’s a cat’ if it’s at a confidence of 85, or 90, whatever produces your preferred response metric.
So given what we know about how the model works, here’s the crucial question: What is it confident about? It doesn’t know what a cat or a question is, only statistical relationships found between data nodes in a training set. A minor tweak would have the cat detector equally confident the picture showed a cow, or the sky, or a still life painting. The model can’t be confident in its own ‘knowledge’ because it has no way of actually evaluating the content of the data it has been trained on.
The AI is expressing how sure it is that its answer appears correct to the user.
This is true of the cat detector, and it is true of GPT-4 — the difference is a matter of the length and complexity of the output. The AI cannot distinguish between a right and wrong answer — it only can make a prediction of how likely a series of words is to be accepted as correct. That is why it must be considered the world’s most comprehensively informed bullshitter rather than an authority on any subject. It doesn’t even know it’s bullshitting you — it has been trained to produce a response that statistically resembles a correct answer, and it will say anything to improve that resemblance.
The AI doesn’t know the answer to any question, because it doesn’t understand the question. It doesn’t know what questions are. It doesn’t ‘know’ anything! The answer follows the question because, extrapolating from its statistical analysis, that series of words is the most likely to follow the previous series of words. Whether those words refer to real places, people, locations, etc. is not material — only that they are like real ones.
It’s the same reason AI can produce a Monet-like painting that isn’t a Monet — all that matters is it has all the characteristics that cause people to identify a piece of artwork as his. Today’s AI approximates factual responses the way it would approximate ‘Water Lilies.’
Now, I hasten to add that this isn’t an original or groundbreaking concept — it’s basically another way to explain the stochastic parrot, or the undersea octopus. Those problems were identified very early by very smart people and represent a great reason to read commentary on tech matters widely.
But in the context of today’s chatbot systems, I’ve just found that people intuitively get this approach: The models don’t understand facts or concepts, but relationships between words, and its responses are an ‘artist’s impression’ of an answer. Their goal, when you get down to it, is to fill in the blank convincingly, not correctly. This is the reason why its responses fundamentally cannot be trusted.
Of course sometimes, even a lot of the time, its answer is correct! And that isn’t an accident: For many questions, the answer that looks the most correct is the correct answer. That is what makes these models so powerful — and dangerous. There is so, so much you can extract from a systematic study of millions of words and documents. And unlike recreating ‘Water Lilies’ exactly, there’s a flexibility to language that lets an approximation of a factual response also be factual — but also make a totally or partially invented response appear equally or more so. The only thing the AI cares about is that the answer scans right.
This leaves the door open to discussions around whether this is truly knowledge, what if anything the models ‘understand,’ if they have achieved some form of intelligence, what intelligence even is and so on. Bring on the Wittgenstein!
Furthermore, it also leaves open the possibility of using these tools in situations where truth isn’t really a concern. If you want to generate five variants of an opening paragraph to get around writer’s block, an AI might be indispensable. If you want to make up a story about two endangered animals, or write a sonnet about Pokémon, go for it. As long as it is not crucial that the response reflects reality, a large language model is a willing and able partner — and not coincidentally, that’s where people seem to be having the most fun with it.
Where and when AI gets it wrong is very, very difficult to predict because the models are too large and opaque. Imagine a card catalog the size of a continent, organized and updated over a period of a hundred years by robots, from first principles that they came up with on the fly. You think you can just walk in and understand the system? It gives a right answer to a difficult question and a wrong answer to an easy one. Why? Right now that is one question that neither AI nor its creators can answer.
This may well change in the future, perhaps even the near future. Everything is moving so quickly and unpredictably that nothing is certain. But for the present this is a useful mental model to keep in mind: The AI wants you to believe it and will say anything to improve its chances.
Reference: https://techcrunch.com/2023/04/03/the-great-pretender/
Ref: techcrunch
MediaDownloader.net -> Free Online Video Downloader, Download Any Video From YouTube, VK, Vimeo, Twitter, Twitch, Tumblr, Tiktok, Telegram, TED, Streamable, Soundcloud, Snapchat, Share, Rumble, Reddit, PuhuTV, Pinterest, Periscope, Ok.ru, MxTakatak, Mixcloud, Mashable, LinkedIn, Likee, Kwai, Izlesene, Instagram, Imgur, IMDB, Ifunny, Gaana, Flickr, Febspot, Facebook, ESPN, Douyin, Dailymotion, Buzzfeed, BluTV, Blogger, Bitchute, Bilibili, Bandcamp, Akıllı, 9GAG