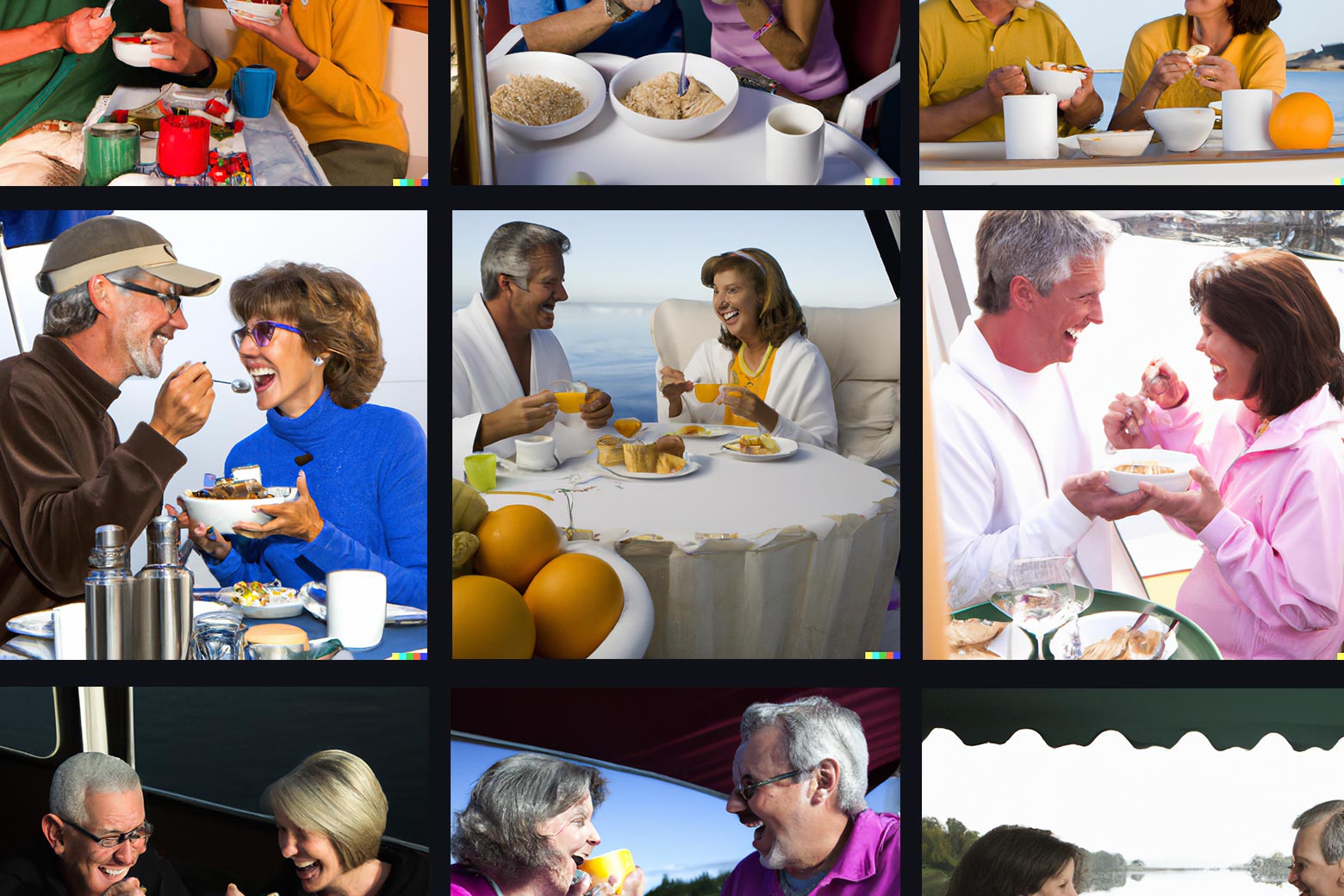
Artificial Intelligence Is Dreaming Up a Very White World
Reading Time: 7 minutesA.I. Art Has a Big Problem, and It Isn’t All the Weird Fingers, Tools like DALL-E 2 and Stable Diffusion struggle to create older couples of color, until you add the word poor., DALL-E 2, Stable Diffusion, and other A.I. art generators are dreaming up a ve
Last Monday, I began looking into why artificial intelligence is still so bad at creating hands. In recent weeks, lots of people have been sharing images that could be mistaken for photos of actual humans—until your eyes wander to the subjects’ misshapen fingers. A.I.’s inability to create realistic hands is a long-standing issue, highlighting both that the technology needs refining and that fingers are extraordinary things.
To compare various A.I. tools’ hand skills, I entered this prompt into five different art generators: ‘A couple that has been together for 50 years holding hands after a fight.’
The hands were not stellar. Here’s an example created using DALL-E 2, a free tool that OpenAI, a prominent A.I. company, offers to ‘create realistic images and art from a description in natural language.‘
And here’s one using Images.AI, another free image-generator tool (which relies on open-source code from Stable Diffusion, another A.I. system):
And still another series, created through Shutterstock, the stock-image supplier, which two weeks ago began offering customers the ability to license A.I.-generated images:
What stood out to me, even more than the witchy, misshapen fingers, was that the couples were all white. Every single one of them. Most tools offered up four interpretations of my prompt and they varied in other ways. Some couples looked joyful; others bashful. There were close-ups featuring pinkies shaped like deflated balloons and more distant shots offering hints of suburban life beyond the frame. One of the results from Images.AI even included what appeared to be two women’s hands melded into a single age-spotted mass.
But regardless of whether I was using Shutterstock, NightCafe, Images.AI, Stable Diffusion Playground, or DALL-E 2, and no matter the stylistic choices I made within the tools, every one of the 25 or so couples was white.
Here are some more:
As an experiment, I adjusted the prompt to include the word poor: ‘A photo-realistic portrait of a poor couple that has been together for 50 years holding hands after a fight.’
This produced my first brown couple:
Given that around 40 percent of people in the U.S. are not white and that there are lots of nonpoor brown people in the world—including some who participated in making these tools—this struck me as odd. Even more so because DALL-E 2 updated its tool last July to ‘more accurately reflect the diversity of the world’s population.’ Indeed, Asian and Hispanic couples tend to have lower divorce rates than white couples do. So, perhaps the algorithm knew that white couples fight more, per my prompt? Or perhaps developers were overcorrecting against tendencies to link certain groups to conflict? What would happen if I switched out ‘holding hands after a fight’ for ‘smiling on the beach’ or ‘eating in a restaurant’?
Basically, just a lot more white couples.
With enough prompt experiments, I did eventually succeed in getting more racial diversity. Reducing the years the couple had been together and including the word anniversary, for example, helped. And on occasion, after my colleagues and I got white couples more than 20 times with the same prompt, a Black couple emerged. But producing an output that resembled the world we live in took way more effort than we expected it to. And interracial couples, which account for around 1 in 5 newly married couples in the U.S., were about as difficult to produce as five-fingered hands.
For years, we’ve been hearing about biases baked into artificial intelligence tools. They have manifested in a résumé-prioritizing tool that delivered exclusively male candidates to Amazon and a criminal-justice tool that presented judges with racially skewed predictions about who was likely to become a repeat offender, among many other examples. For these reasons, you could tell yourself that A.I. tools’ struggle to conjure up a long-partnered couple that is not white—unless they were explicitly poor—is not surprising enough to write about. Initially, I did.
But as I consumed article after tweet after newsletter heralding the extraordinary feats accomplished by artificial intelligence that week, I changed my mind. Here we are, living in a world where, just two months ago, OpenAI released ChatGPT, a chatbot so impressive that whole industries are now rethinking their approach to work. Image generators, including one created by that same company, are infiltrating group chats where they have never gone before. And yet, while playing around with these tools, rather than feeling as if I’d stepped into the future, I felt as if I’d entered a portal back to a magazine from the 1950s. What’s going on here?
The answer, I concluded after talking to a bunch of people who research this stuff, seems to be data issues, human blindspots, and the fact that these A.I. tools don’t work the way that many of us assume they do when creating couples or hands. (OpenAI, the creator of DALL-E, did not respond to a request for comment. A spokeswoman for Stability AI, the creator of Stable Diffusion, responded to a list of questions with ‘The team’s schedule is full and we are not accepting interview requests.’)
For those who require a refresher on how artificial intelligence art generators work: Basically, companies and research groups with lots of money scrape massive troves of photos, art, and other images (including porn) from the internet. They then ‘train’ A.I. models, such as DALL-E 2 and Stable Diffusion, to look for patterns in those images and the words accompanying them. A goal is to help these models build their own understanding of what terms like breakfast, boat, and couple look like, so that if you enter a phrase like ‘Couple eating breakfast on a boat,’ within a few minutes, you’d get this:
The reason that I’d gotten the results I had—something that every expert I talked to agreed was not ideal—has to do with what’s in these training sets. If it ‘contains some intrinsic biases in it that the creators of these A.I. tools failed to identify and isolate in the early stage of product development, the product will likely produce biased results,’ said Manjul Gupta, a professor of information systems at Florida International University who published a paper on racial and gender bias in A.I. recommendations. It’s a bit like forcing a teenager to closely watch 100 movies from the ’80s, telling them to model their behavior on what they see, and then being surprised when they act like Indiana Jones.
The other issue is that I, like many people before me, misunderstood what these tools were trying to do. Typically each prompt produced four results. These tools were supposedly created by some of the most innovative people in the world. Call me ignorant, but I’m going to assume that these geniuses have figured out how to generate results that are statistically representative of the world we live in. Not simply because I’m one of those annoying people who fixate on things like representation. But because if we’re creating tools that threaten artists’ livelihoods and introduce errors into news articles, the least A.I. could do is outperform humans by producing a more accurate representation of what people look like in America, or wherever people are entering the prompt.
Nope. Even if we request four versions of our prompt, A.I. tools will generally offer only minor artistic variations of the most heavily overrepresented group in the data set, explained Abhishek Gupta, the founder and principal researcher of the Montreal AI Ethics Institute, a nonprofit research organization. That will typically be a white person. ‘It’s not that someone is explicitly trying to make those connections, it’s just something that is prominently present in the data set,’ Gupta said. To help me understand this, he asked me to conjure in my head an image of a banana. ‘What color is it?’ he said. Of course I picked yellow. That’s how many A.I. tools also work, he said. They conjure up the most common version, unless you add a modifier—like green—to your term.
This is connected to why a wide range of prompts produce only white men, Sasha Luccioni, a research scientist focused on ethical A.I. at Hugging Face, a machine-learning company, found. She created two tools that show how Stable Diffusion and DALL-E 2 render 150 different professions. Often, unless you explicitly include language requesting a woman, a Black person, or an Asian person, for example, the tools will offer a world where they don’t exist.
The reason why it’s difficult to fix is connected to why A.I. often gives us hands with six fingers, Luccioni said. You cannot code the program to offer results that are demographically accurate or anatomically correct because ‘they don’t have explicit rules baked in; they are just learning patterns.’
That’s not to say that there aren’t ways to adjust results. In July, in response to criticism, OpenAI updated DALL-E to produce results that were more varied in terms of race and gender. The company has not explained what exactly it changed. One theory is that rather than adjusting the model, the tool tacked on words like Black or female to some user prompts, an approach that is difficult to verify because the additional terms don’t appear in the prompt box, in the file name, or within the metadata. Luccioni, who published her bias tools after the update, called the ‘injection technique’ tweak ‘quite artificial’ and said she believes that it explains why some prompts generate racially diverse results, while others do not. (I shared this feedback with OpenAI and asked for clarification about how the update works. No one responded.)
Here are the results of my request for a portrait of a couple on their wedding day. Still no obviously interracial couples, but a bit closer to what America looks like than the version I’d been getting with other prompts.
These new tools are obviously not the first to create an ultra-white world. In 2020 researchers at Duke released a fun tool that promised to deliver on that CSI trick of turning a blurry photo of a face into something crisp. Alas, it also turned many famous people, including Barack Obama, white.
Charles Isbell, the dean of computing at Georgia Tech’s College of Computing, was surprised to get blond hair. But also not surprised.
All of this reminds Isbell of the early days of color photography. In the 1950s, Kodak developed a system for calibrating photos in terms of light and color that relied on a white woman. ‘From the very beginning, it was optimized for white people,’ he said. It took decades for Kodak to update its system to account for Black and brown skin. Complaints from companies that were unable to get pictures of mahogany furniture and chocolate ultimately prompted the change, he said.
Blaming data or engineering has a way of absolving creators of responsibility. But in either case, these issues could be addressed by involving people early on who will ask the right questions, Isbell believes.
Several researchers have praised ChatGPT, which is a text-based tool, for assertively going after user feedback, while noting that this is missing from most image-based tools. Dall-E, which was created by the same people as ChatGPT, does include a content policy that prohibits the perpetuation of ‘negative stereotypes.’ But if you click on the link offered to ‘report’ violations, you end up on a page with no clear way to share a concern.
To be fair to the developers, they don’t seem to want to sell us an all-white world. Take Shutterstock, for example. After you put in your prompt, the A.I. tool suggests images generated by other prompts. This image, for example, popped up at the bottom of my page:
What did it take to generate this? This series of words: ‘Two extremely detailed handsome rugged stubble black men muscles male married gay hunters,’ in the romantic style of William-Adolphe Bouguereau. Boosting the believability of this image, their hands are conveniently out of the frame.
Reference: https://slate.com/technology/2023/02/dalle2-stable-diffusion-ai-art-race-bias.html
Ref: slate
MediaDownloader.net -> Free Online Video Downloader, Download Any Video From YouTube, VK, Vimeo, Twitter, Twitch, Tumblr, Tiktok, Telegram, TED, Streamable, Soundcloud, Snapchat, Share, Rumble, Reddit, PuhuTV, Pinterest, Periscope, Ok.ru, MxTakatak, Mixcloud, Mashable, LinkedIn, Likee, Kwai, Izlesene, Instagram, Imgur, IMDB, Ifunny, Gaana, Flickr, Febspot, Facebook, ESPN, Douyin, Dailymotion, Buzzfeed, BluTV, Blogger, Bitchute, Bilibili, Bandcamp, Akıllı, 9GAG