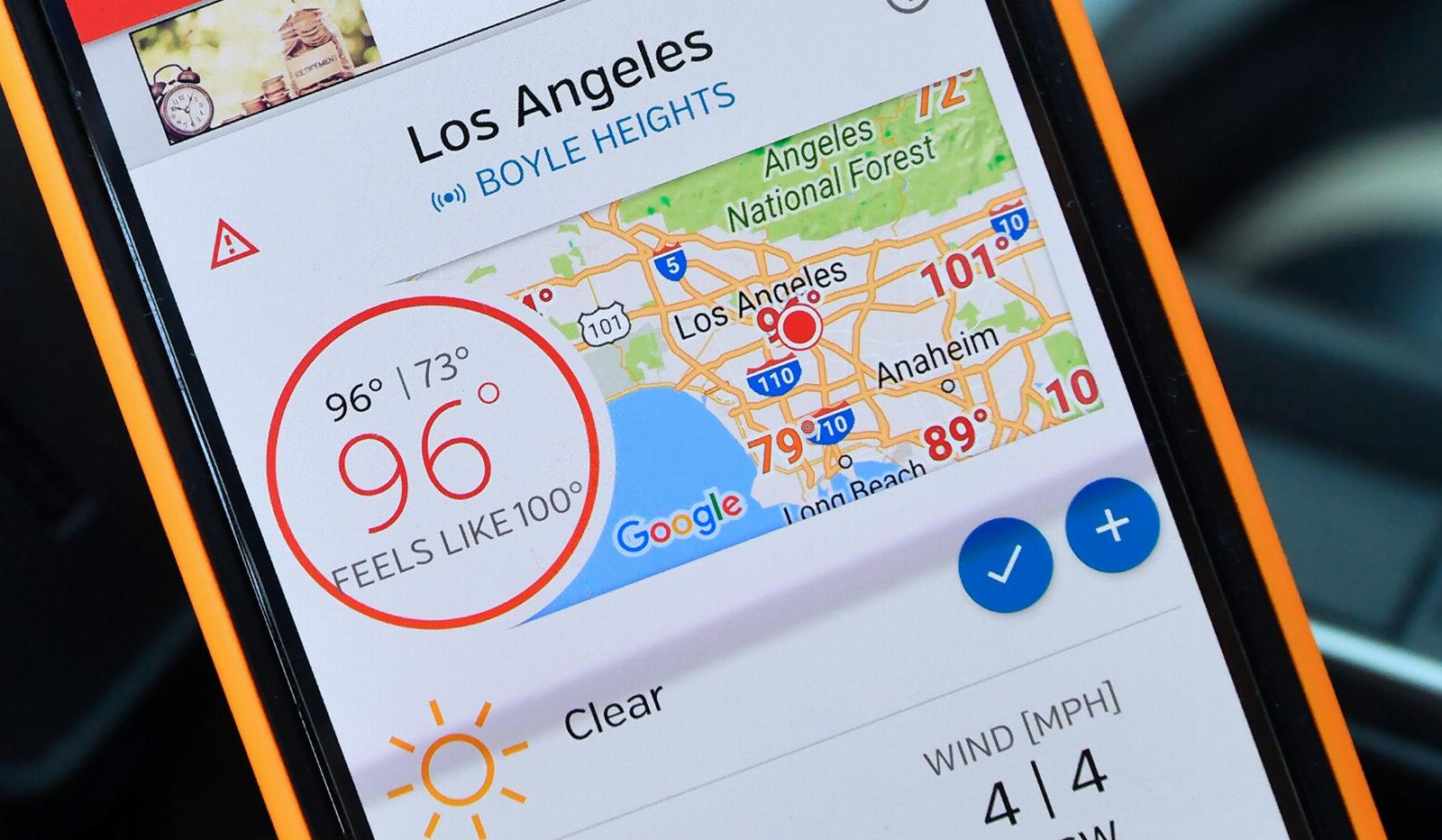
What Real Meteorologists Wish You Knew About Your Weather App
Reading Time: 6 minutesWhy weather apps are so unreliable.
Most of us don’t have a favorite TV meteorologist anymore. Instead, we roll out of bed and check a weather app on our phones. The problem? Most weather apps kind of suck. When they fail—and they often do—we feel confused and almost betrayed. After all, we chose this weather app, checked it days in advance, trusted it with our plans … and the prediction it made led us astray, maybe left us soaking wet.
Many professional meteorologists call weather apps ‘crap apps.’ And as climate change accelerates extreme weather events, their consequences are much bigger than soggy T-shirts.
On a recent episode of What Next: TBD, I talked with Daniel Swain, a climate scientist at UCLA, about how apps have transformed the way we interact with weather forecasts.
Our conversation has been edited and condensed for clarity.
Lizzie O’Leary: Why are weather apps so bad?
Daniel Swain: Well, one is that these apps lack context. What exactly is being predicted by these apps in any given place or at any given time? One thing that people really notice is how radically the forecast sometimes changes from one hour to the next when you look at the app.
So if you’re looking at the forecast for four or five days from now, for example, you might check in the morning when you get up, it might be a relatively warm and clear forecast. And then six hours later you check and the forecast is now cold and rainy. You might say, ‘How the heck did it change that much in six hours? You can’t really rely on anything these days.’ But the reality is the actual prediction didn’t change that much in six hours. What happened is that this app in this example is only looking at one of very many realizations across an ensemble of weather model predictions for that same period.
The realization you are seeing is one possible outcome of a model that gets run every six hours. But weather is more complex. It’s a broader envelope of outcomes, which you’re probably not seeing in your app. How does that impact an app’s accuracy?
If you look at the whole envelope, the full ensemble, the full distribution of possible weather futures, it’s likely that it didn’t change very much. The app is inadvisably picking only one member and giving you a prediction that’s uncorrected by human forecasting expertise. That’s not a statistically rigorous way of assessing things, and it’s not giving you a contextually meaningful view of how much the actual likely future outcome has changed over that six-hour period. So it’s literally true, but contextually missing the point.
What other factors make it difficult to create an accurate weather forecast?
We don’t know exactly what the world’s atmosphere looks like in this moment right now. Imagine you were to push ‘go’ on one of these mathematical weather models. When you run a prediction, you need some sense of what the initial conditions are, what’s the starting point that you’re predicting from. But we don’t know that initial starting point precisely. There’s actually quite a bit of uncertainty, because weather models need to know everything about the entire vertical 3D structure of the atmosphere over the whole world to make multi-day weather predictions. So you need to know what the wind speeds are 500 feet off of the ocean in the remote South Pacific, for example, along with everywhere else. And we don’t have that information precisely. We can estimate it within certain reasonable bounds of uncertainty using observations and satellites and interpolations. But as you’d expect, all of those are imprecise. And so if you get it wrong, then you’re going to get a forecast that is imperfect.
The way to make these forecasts better is to use ensembles to run the weather model dozens of times with slightly different conditions. The more times you run it, the more accurate your forecast will be. What does that process look like?
So you feed the model, over and over again, slightly different sets of initial conditions, all of which are plausible given that we don’t know exactly what the real world is. You run it and you get 20, 30, 40 different plausible futures in terms of what might happen to the weather in three to five days. Because of that, any individual member of that ensemble might do something weird if those initial conditions produce some weird outcome. The most realistic way to interpret these ensembles is to look at all of the members collectively and look at trends and patterns across all of them. The challenge is a lot of these weather apps are just picking one.
Do any apps look at the whole ensemble?
Some do, but one challenge is that where the data is coming from and how these forecasts are generated in these proprietary weather apps is very opaque. I actually tried to look at the sourcing for some of the most popular weather apps, and they claim to be transparent, but from a scientific perspective, I have no idea how these apps are actually assimilating the data.
As a society we have put our faith in these microcomputers we carry around. Listening to you, it’s very clear that actual human skill in differentiating between and interpreting these bands of probability might be a better predictor of telling me what to wear in the morning.
My go-to in the U.S. is the National Weather Service, weather.gov. There are local weather forecast offices with real human meteorologists living in a particular part of the country who specialize in predicting the weather for that region. And they know all of the local microclimates, the quirks of weather in your area, including understanding the kinds of regionally specific problems that existing numerical weather models might have. And so all of that human knowledge is actually quite substantial in producing what I think are much more nuanced and better forecasts.
This human knowledge is the kind of thing that a lot of artificial intelligence and machine learning folks are trying to get generative A.I. models to assimilate, but we haven’t gotten there yet. We just don’t have numerical tools that replace the human touch in this context. We really do need domain-specific knowledge to contextualize these huge arrays of raw numbers that are thrown at us from predictive models like weather models. Human meteorologists probably aren’t going to be out of a job anytime soon.
Thinking about what you do, which is looking at this intersection between climate change and extreme weather, I wonder if there’s something even more immediate about understanding why people need reliable weather and climate-related information that they can use to make decisions?
It’s a huge challenge. There is this assumption that because we have this powerful computer in our pocket, the basic day-to-day information that’s delivered to us, like a weather forecast, must be correct. And I think that sort of lends folks, in some cases, a bit of a false sense of security.
One example might be, if you just look at the icon in your weather app and you’re in the central United States during tornado season, depending on how the app interprets the forecast, there might only be a 20 or 30 percent chance of isolated thunderstorms. And so the app might give you essentially a warm and partly cloudy icon, with a little 20 or 30 percent mentioned in the corner. But the reality is that if you are under one of those areas covered by thunderstorms, they might be really violent storms, there might be tornadoes. And so the single icon … it’s a science communication choice. Do you emphasize the low but actually quite consequential risk of severe weather in this kind of forecast? Or do you emphasize what the weather is going to be like most of the time that day, which is probably warm and partly cloudy until the isolated tornadic thunderstorms arrive?
We are seeing more extreme heat waves and more extreme downpours in a warming climate. More and more people are experiencing conditions that are certainly outside their realm of personal experience and increasingly also outside the realm of historical experience. Properly communicating weather, and particularly extreme weather, in a climate change context is only becoming more important. And so we have this simultaneous reality of unprecedented instantaneous potential access to information, but for a lot of folks the context of that information has perhaps gotten worse. Because in the past, you might have tuned in to your local morning news station and there would’ve been an on-air meteorologist who actually did some of that interpolating for you, whereas now, increasingly you’re just sort of getting the raw, unfiltered version without any framing.
Can you envision a better weather app?
Honestly, I haven’t seen it yet, and it’s one of the reasons why I personally tend not to use weather apps as a professional atmospheric scientist. I do think there are a couple that are probably somewhat better than others, but in general, it’s difficult—because it is a science problem, a science communication problem, and a technology user-interface problem. And I’m not sure that we have great solutions for any of those three things in this context yet. It’s important to have domain experts on board, because a lot of the folks producing weather apps don’t necessarily have a background in atmospheric science or meteorology. That’s not universally true, but there is so much knowledge among folks who’ve been doing this for a long time—and that just doesn’t make it into the current system.
Reference: https://slate.com/technology/2023/05/weather-apps-climate-change.html
Ref: slate
MediaDownloader.net -> Free Online Video Downloader, Download Any Video From YouTube, VK, Vimeo, Twitter, Twitch, Tumblr, Tiktok, Telegram, TED, Streamable, Soundcloud, Snapchat, Share, Rumble, Reddit, PuhuTV, Pinterest, Periscope, Ok.ru, MxTakatak, Mixcloud, Mashable, LinkedIn, Likee, Kwai, Izlesene, Instagram, Imgur, IMDB, Ifunny, Gaana, Flickr, Febspot, Facebook, ESPN, Douyin, Dailymotion, Buzzfeed, BluTV, Blogger, Bitchute, Bilibili, Bandcamp, Akıllı, 9GAG