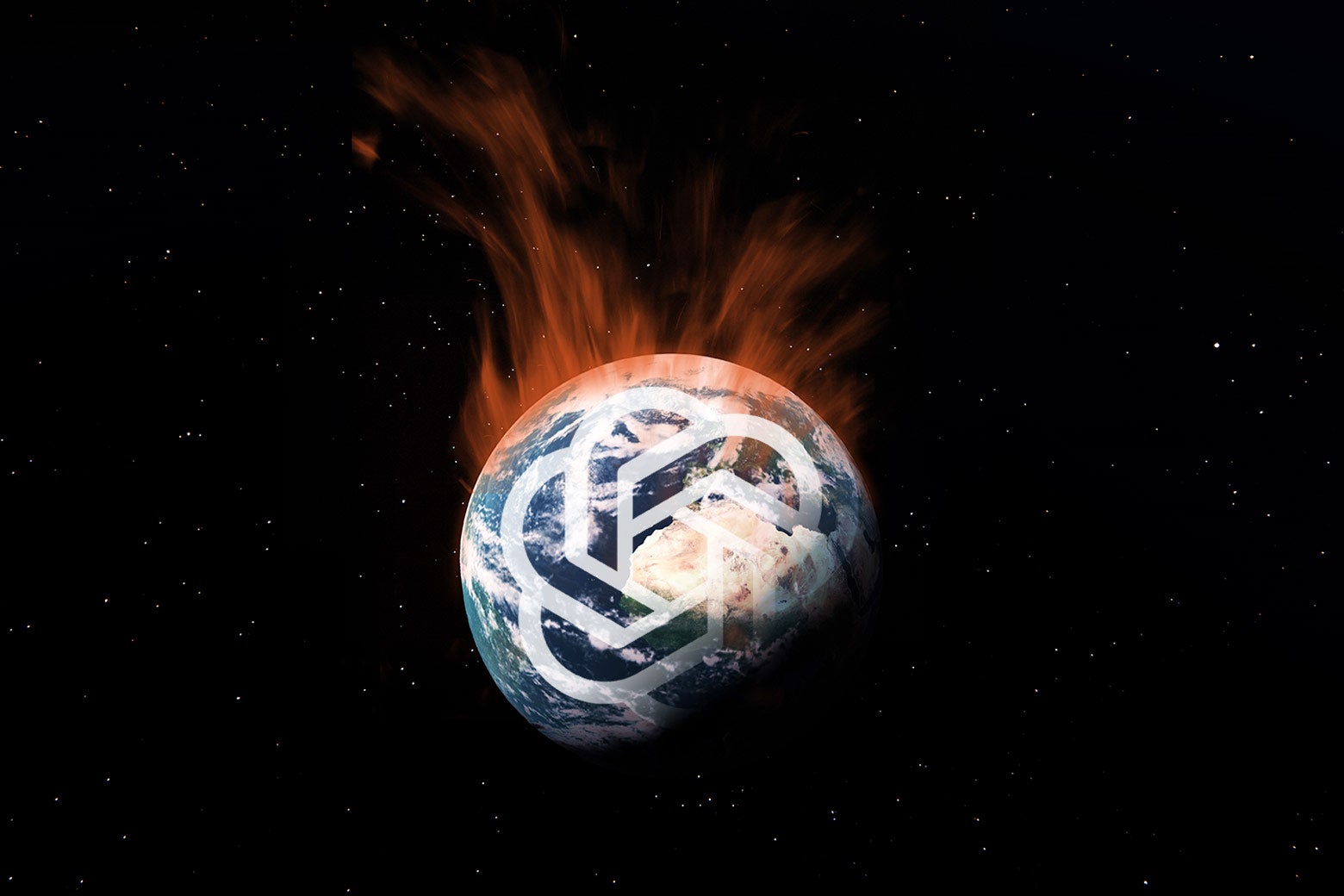
Is the A.I. Arms Race Unsustainable?
Reading Time: 9 minutesThe Risky Assumption Propping Up the A.I. Arms Race, A.I. tunnel vision could have disastrous consequences for the climate., A.I. and climate change: Why A.I. tools like ChatGPT use so much energy.
There’s a big reason why every company hoping to deal in some way with artificial intelligence is either spending or raising billions upon billions of dollars right now, and it’s not just investor hype. These stacks of cash are necessary for meeting the costs of building, training, and maintaining resource-intensive (and resource-lacking) power-sucking content generators like ChatGPT, as well as the resource-intensive power-sucking data sets, neural networks, and large language models, or LLMs, they’re trained on—such as OpenAI’s GPT-4, whose API was recently made public to paying customers with coding expertise.
Someone who understands the energy issue all too well is OpenAI’s CEO himself, Sam Altman. Back in May, while testifying to Congress about the challenges wrought by the A.I. arms race his company ushered into the world, Altman admitted something curious: that he’d prefer for his wildly popular ChatGPT bot, at that time the fastest-growing app in history, to have fewer users. ‘We’re not trying to get them to use it more,’ he stated. ‘Actually, we’d love it if they use it less, because we don’t have enough GPUs.’
With ‘GPUs,’ Altman was referring to graphics processing units—the specialized power-intensive processors used to render images in video games, mine Bitcoins, and power various types of A.I. Due to the mass popularity of all three of these sectors, affordable GPUs are hard to find, companies run by A.I.-curious executives like Mark Zuckerberg and Elon Musk are stocking up on large GPUs at their corporations, and investors are searching for chip manufacturers that can crank out enough units to support the demand.
The collective demand for GPUs has escalated such that Nvidia will be sold out of treasured units like its H100 through the rest of the year. In the meantime, some cryptocurrency enthusiasts are repurposing their power-draining mining machines for the A.I. training cause, and Google is banking on its TPUs (tensor processing units, which were invented by Google specifically to handle compute requirements for machine learning tech).
Even before the demand for GPUs skyrocketed, the technology wasn’t cheap. Earlier this year, Altman admitted to a fellow A.I. exec that a ‘huge margin‘ of OpenAI’s expenses involved ‘compute,’ defined as the technical resources required to train, tweak, and deploy LLMs. In 2018, OpenAI published a now frequently cited report titled ‘A.I. and Compute,’ noting that ‘since 2012, the amount of compute used in the largest AI training runs has been increasing exponentially’ and pinpointing how ‘more compute seems to lead predictably to better performance.’ The post further notes that ‘we believe the largest training runs today employ hardware that cost in the single digit millions of dollars to purchase,’ including GPUs and TPUs. And, as stands to reason, advanced A.I. models not only used hundreds of such units, but they also employed higher-performing versions of these models.
In other words: The stuff that allows ChatGPT to pen inadmissible legal briefs and error-laden blog posts in mere seconds uses a lot of hardware that transmits a lot of electricity. And if these tools are any good at the moment, it’s because the data sets on which they’re trained are only getting bigger and bigger—and the physical infrastructure that they run on has to bulk and scale up as well.
Unsurprisingly, then, ‘the compute costs are eye-watering‘ when it comes to A.I.
development, as Altman tweeted in December, explaining to an enthusiastic user why the mostly free-to-use ChatGPT would have to be ‘monetized.’ Altman has been well attuned to this fact for a while, and has been remarkably unshy about calling it out. ‘The compute costs get non-trivial for us,’ he told a tweeter last August, laying out why OpenAI’s image-generating DALL-E 2 did not have a more ‘generous’ pricing plan just yet.
This is key to understanding why the A.I. sector looks the way it does: It’s primarily controlled by Big Tech corporations that own varied and ample resources, dependent on large and consistent cash influxes, hopeful about long-evasive moonshots in fields like quantum computing and nuclear fusion, dismissive of smaller competitors who can’t hope to catch up to the bigger firms’ staggering advancements, secretive about the technical factors behind its energy inputs.
Even Andreessen Horowitz, the venture capital firm whose founders are megabullish on the A.I. future, has admitted that ‘access to compute resources—at the lowest total cost—has become a determining factor for the success of AI companies. … In fact, we’ve seen many companies spend more than 80% of their total capital raised on compute resources!’ Here, OpenAI has a huge advantage over any upstart competitors thanks to billions of dollars of investment from Microsoft, in addition to that company’s willingness to shell out stacks on stacks for exclusive custom supercomputers.
With greater power has come reduced transparency. GPT-4’s API is visible to more of the world, but public knowledge about its workings remains limited: When OpenAI’s report on the model came out in March, it controversially excluded ‘further details about the architecture (including model size), hardware, training compute, dataset construction, training method.’
Nonstop fear over Singularity-esque robohuman consciousness often fails to take the very real physical limits of today’s A.I. into account—and, resultingly, its very real impact on the planet. We know much less about that than we should, even as we currently endure record high temperatures fueled by climate change. It’s not that A.I.’s carbon footprint hasn’t been studied or warned about: In 2019, my former colleague April Glaser interviewed a researcher who’d co-published a prominent academic paper that year about A.I.’s climate effects. But that same paper, titled ‘Green A.I.,’ continues to be the main source on which tech reporters rely, up through today, to quantify the A.I.-climate issue. Needless to say, a lot has changed in the four years since, in terms of tech capabilities, investment, and energy efficiency (or lack thereof).
So, with OpenAI and other big-name players like Google refusing to share details that could inspire scrutiny over their A.I. energy use and environmental fallout, how should we perceive the technology’s ever-advancing capabilities and their contributions to climate change? To answer this question, let’s break down the exact components of what we do know about how ChatGPT functions.
First, let’s look at the backbone signified by the ‘GPT’ acronym: a Generative Pre-trained Transformer. The ‘Transformer’ signified here is ‘a novel neural network architecture based on a self-attention mechanism‘ that was invented by Google in 2017. A neural network is, in very simple terms, a technical model formed by interconnecting a bunch of ‘nodes’—basically, individual mathematical functions—in an arrangement meant to resemble that of the human brain. (Don’t worry about it.)
Neural networks have been around for a while, but what makes the Transformer unique is that, per Google, when it comes to detecting language patterns and contexts, it ‘requires less computation to train’ than previous types of neural networks. You could feed a Transformer a lot more information than prior neural models by feeding in units of data known as ‘tokens’—which the network can process, understand, and memorize in an economical manner—while using much less energy, time, and money than what less-slick neural networks may require. This is why current A.I. models have better predictive and generative capabilities: Many are now trained on hundreds of billions of these tokens, which thus establishes billions of ‘parameters,’ aka the ‘synapses’ of neural networks (more on that later).
That’s the T, but what about the GP? The ‘Generative Pre-trained’ innovation is what OpenAI had added to Google’s invention by 2018. ‘Pre-trained’ refers to how the OpenAI Transformer has been fed a particular data set—in GPT models’ instance, troves of text scraped from books and webpages—that the system processes in order to establish itself as ‘learned’ in various language patterns and contexts, denoted in parameters. ‘Generative’ refers to these models’ capability to, naturally, generate text that’s (often) legible and (sometimes) sensible, based on what they’ve been pre-trained on through the Transformer.
Every part of this process requires ample energy. Some academics, when discussing the carbon footprint of A.I., focus on all parts of developing the tech—everything from sourcing the materials required to shipping them through supply chains to the flights that individual A.I. researchers take to collaborate with one another or gather at conferences. But, for our purposes, let’s keep it simple and focus on the process that occurs from text system training to final output, as tested and deployed in a laboratory that has the pieces assembled and ready. (To get into image, video, and audio generation would require a more elaborate breakdown.)
First, the data. In A.I., much text data is scraped online from various websites (like MediaDownloader) in a bulk-collection method that often spikes the number of requests sent to a given site and can overwhelm its servers—in effect, outsourcing the energy usage to the millions of sites being scraped. The scraped data must be stored somewhere; Microsoft and other companies delving further into A.I. are constructing bigger ‘hyperscale‘ data-center campuses, often in big cities or in European regions with colder weather, the latter providing the advantage of naturally moderating the operational temperatures of these data centers.
The necessity of constantly running, maintaining, and stabilizing these data centers releases hundreds of metric tons of carbon emissions. In hot climates, cooling non-A.I. data centers requires billions of gallons of water. The tech analysis firm TIRIAS Research estimates that global data-center power consumption could increase by 21,200 percent in five years, running up operational costs in excess of $76 billion (in today’s dollars). To meet this skyrocketing energy demand in a sustainable manner, we’re gonna need a lot more renewable energy.
There’s the matter of keeping the data you’ve scraped on deck and ready at all times.
And then there’s the process of actually training your neural network, which you’d like to become as big as possible, perhaps including trillions of nodes and parameters and interconnected layers. Why so huge? Because, as OpenAI noted in the aforementioned 2018 report, the bigger the model, the faster and more accurate its output will be—or at least, that’s what OpenAI’s track record seems to demonstrate, from its very first GPT model through its current GPT-4 iteration.
As researchers from Meta and from academia noted in a paper from May, ‘large language models are trained in two stages: (1) unsupervised pretraining from raw text, to learn general-purpose representations, and (2) large scale instruction tuning and reinforcement learning, to better align to end tasks and user preferences.’ In other words: There’s the first step of shoveling in mounds of data that the model grows and learns from, and then there’s the question of further tweaking the model after the first ‘pre-training’ is complete.
This includes refining and expanding the model after the fact, through processes like fine-tuning and reinforcement learning from human feedback, or RLHF. The former refers to the technical practice of adding more real-word-example data for the LLM’s benefit, so that it establishes a wider berth of knowledge without starting training all over again; RLHF is the means by which a human content trainer assists training, whether by grading certain bits of output or feeding refined data that will (hopefully) help to produce a desired result. For example: You know how you ask ChatGPT all your stupid little questions and you either 1) click the thumbs-up or thumbs-down icon depending on what you receive, or 2) tell ChatGPT explicitly that it did something right/wrong and offer it a way to correct itself? That’s RLHF in action, baby, outsourced all the way to your desktop or phone.
Fine-tuning takes place at the research and development end, but RLHF has more reach: It’s the masses of underpaid workers labeling bits of data to make it easier for the computer to learn factual things, and us humans telling ChatGPT why its summary of energy history was wrong, wrong, wrong. In fact, much of the reason ChatGPT existed in the first place was so that OpenAI could hasten the improvement of the model it was working on—in the chatbot’s case, GPT-3—and take it to the next level.
But when it comes to making ChatGPT more competent, drawing on willing volunteer trainers isn’t an automatic cost-cutter. Unlike fine-tuning, which directly futzes with the mechanics of a neutral network, 100 million users doing RLHF means that the model is also being simultaneously deployed for use—it’s being applied to the real world, through an action known as ‘inference.’
GPTs may have their pre-training, but they still require compute and energy to spit out answers and paragraphs upon prompting. As the semiconductor research and consulting firm SemiAnalysis reported, ‘inference costs far exceed training costs when deploying a model at any reasonable scale. In fact, the costs to inference ChatGPT exceed the training costs on a weekly basis.’ Per SemiAnalysis’ own calculations, ‘ChatGPT costs $694,444 per day to operate in compute hardware costs,’ equating to about 36 cents per interaction.
All of that is on top of the cost it took merely to prepare ChatGPT as you know it. According to A.I. analyst Elliot Turner, the compute cost for the initial training run alone probably summed up to $12 million—200 times the cost of training GPT-2, which only had 1.5 billion parameters. In early 2021, researchers from Google and the University of California–Berkeley estimated that merely training GPT-3 consumed up to 1,287 megawatt-hours of electricity, enough to power about 360 homes for a year—all before you get into the inference. And this is all for text output, mind you; the energy and emissions tolls go way up when you get into image and video generation.
Mapping all this out helps us understand the sheer amount of monetary and physical resources needed if A.I. will supposedly control the future.
For many developers, the goal now is to ensure that generative A.I. does not need to rely on such hulking infrastructure. Researchers at Stanford are working to develop advanced neural models that could be even more power-efficient than Transformers when it comes to training and deployment. Google and Meta are hoping that advanced enough pre-training for LLMs can reduce the need for further intensive fine-tuning, making deployment much cheaper and more accessible on smaller forms of hardware. Different parts of the A.I. power process—location and efficiency of data centers, improvements in neural-network architecture, shortcuts in training, sourcing of compute electricity from solar, wind, and nuclear hookups, or from grids powered by renewables—can be tweaked along the way to lessen the impact.
Yet what’s so alarming is that the hype, the competition, the energy, and the money being devoted to A.I. right now threatens to overwhelm and undermine the investments we’re only finally making to mitigate the threats of climate change. We need those energy sources, clean and dirty, for our everyday needs as we transition from fossil fuels to greener power; we need those same semiconductors and chips used in A.I. data centers and computing for clean-energy setups and electric vehicles; we need those landmasses being dedicated to A.I. data centers for agriculture, shelter, and environmental upkeep; we need the water used to cool those data centers for consumption, irrigation, and wildlife protection; we need to ease pressure and demand on our electric grids, which are already overwhelmed thanks in large part to climate change–fueled extreme weather.
In a timeline where humanity had taken earlier, more decisive action to prevent and reduce the harms of global warming, a more sustainable version of this A.I. development race may have been possible. But in a time when the costs of inaction have already contributed to record temperatures, frequent weather disasters, and biodiversity crises that threaten to upend Earth’s ecosystems, the rapid manifestation of this A.I. tunnel vision seems harder to justify.
Reference: https://slate.com/technology/2023/08/chatgpt-ai-arms-race-sustainability.html
Ref: slate
MediaDownloader.net -> Free Online Video Downloader, Download Any Video From YouTube, VK, Vimeo, Twitter, Twitch, Tumblr, Tiktok, Telegram, TED, Streamable, Soundcloud, Snapchat, Share, Rumble, Reddit, PuhuTV, Pinterest, Periscope, Ok.ru, MxTakatak, Mixcloud, Mashable, LinkedIn, Likee, Kwai, Izlesene, Instagram, Imgur, IMDB, Ifunny, Gaana, Flickr, Febspot, Facebook, ESPN, Douyin, Dailymotion, Buzzfeed, BluTV, Blogger, Bitchute, Bilibili, Bandcamp, Akıllı, 9GAG